Featured
- Get link
- Other Apps
Big Data and Analytics in Risk Management: Navigating Financial Markets with Data-Driven Insights
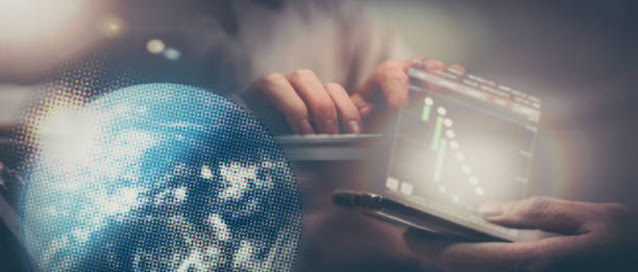
Big Data and Analytics in Risk Management: Navigating Financial Markets with Data-Driven Insights
In the rapidly evolving world of financial markets, the
volume and complexity of data continue to grow. To effectively manage risks and
make informed decisions, institutions turn to big data and advanced analytics.
These tools provide a way to assess, predict, and mitigate risks by uncovering
patterns, anomalies, and insights that might otherwise remain hidden. In this
article, we will explore how big data and advanced analytics are employed in
risk management to navigate the challenges and opportunities in financial
markets.
The Role of Big Data in Risk Management
Big data refers to the vast and varied datasets that are too
large and complex to be processed by traditional data management tools. The
financial industry generates enormous volumes of data daily, from market prices
and trading volumes to economic indicators and news sentiment. Big data in risk
management encompasses several key aspects:
- Data
Variety: Financial data includes structured information like market
prices and unstructured sources such as news articles and social media
sentiment.
- Data
Velocity: Market data is generated in real time, and analytics must
keep pace with the speed at which this data is produced.
- Data
Volume: The sheer volume of financial data requires scalable and
high-capacity storage and processing solutions.
- Data
Veracity: Ensuring data accuracy and reliability is essential for
making sound risk management decisions.
Utilizing Advanced Analytics
Advanced analytics encompasses a range of techniques and
technologies, including statistical analysis, machine learning, artificial
intelligence, and data mining. These tools allow risk management professionals
to extract meaningful insights and patterns from big data, thereby enhancing
decision-making and risk assessment. Here are some key areas where advanced
analytics play a pivotal role in risk management:
- Predictive
Modeling: Advanced analytics can be used to build predictive models
that anticipate future market movements or specific risk events.
- Portfolio
Optimization: Tools like mean-variance optimization help investors
construct portfolios that balance risk and return effectively.
- Credit
Risk Assessment: Machine learning models can evaluate creditworthiness
by analyzing historical data, reducing the risk of loan defaults.
- Market
Risk Analysis: Analytics are employed to model and measure market
risk, enabling institutions to gauge potential losses in various market
conditions.
- Fraud
Detection: Advanced analytics can uncover patterns associated with
fraudulent activities, providing financial institutions with tools to
identify and mitigate risks.
Benefits of Big Data and Analytics in Risk Management
The integration of big data and advanced analytics into risk
management offers several advantages:
- Improved
Risk Assessment: Big data and analytics provide a more comprehensive
view of risk factors, enabling more accurate risk assessments.
- Real-Time
Monitoring: Real-time data analysis allows for immediate responses to
market events, reducing reaction time.
- Identification
of Hidden Patterns: Analytics can reveal hidden patterns and
correlations that may not be apparent through traditional methods.
- Enhanced
Decision-Making: Informed decisions can be made based on data-driven
insights, reducing the reliance on intuition and gut feeling.
- Cost
Reduction: Streamlined processes and automated risk assessments can
result in cost savings for financial institutions.
Risk Management Applications of Big Data and Analytics
Big data and analytics find numerous applications in risk
management in the financial industry:
- Credit
Risk Assessment: Advanced analytics assess the creditworthiness of
borrowers based on historical data, reducing the risk of loan defaults.
- Market
Risk Management: Analytics model and measure market risk, allowing
institutions to understand potential losses under different market
conditions.
- Operational
Risk Analysis: Patterns of operational risk, such as fraud, can be
detected through data analysis, enabling preventive measures.
- Fraud
Detection: Analytics uncover fraudulent activities by identifying
anomalies and unusual patterns in transactions.
- Compliance
Monitoring: Advanced analytics help institutions comply with
regulatory requirements by monitoring transactions and flagging potential
issues.
- Liquidity
Risk Management: Institutions use analytics to assess liquidity risk
and ensure they can meet short-term financial obligations.
Challenges and Considerations
While big data and advanced analytics offer significant
benefits, they also present challenges:
- Data
Privacy and Security: The handling of sensitive financial data
requires robust security measures to protect against breaches and cyber
threats.
- Data
Quality: Ensuring data accuracy and completeness is essential for
reliable analytics.
- Regulatory
Compliance: Compliance with data privacy laws and financial
regulations is critical, especially when handling customer data.
- Resource
Requirements: Implementing and maintaining advanced analytics
solutions can be resource-intensive in terms of technology, personnel, and
infrastructure.
- Interpretability:
Advanced analytics models may be complex and difficult to interpret, which
poses challenges for risk professionals.
- Data
Integration: Integrating data from various sources and systems can be
a complex and time-consuming process.
Case Study: Credit Scoring
One practical application of big data and analytics is
credit scoring. Traditional credit scoring models rely on limited data sources,
such as credit history and income. In contrast, big data analytics allow
lenders to consider a broader range of factors, including:
- Social
media activity
- Online
behavior and e-commerce transactions
- Mobile
phone usage patterns
- Geographic
location and housing data
By analyzing these diverse data sources, lenders can develop
more accurate credit scores, increasing their ability to assess credit risk and
make informed lending decisions.
Conclusion
Big data and advanced analytics have revolutionized risk management in the financial industry. These tools enable institutions to assess, predict, and mitigate risks more effectively by providing a comprehensive view of risk factors and market dynamics. While they come with challenges related to data privacy, quality, and regulatory compliance, the benefits of improved risk assessment, real-time monitoring, and enhanced decision-making make big data and analytics essential for navigating financial markets with confidence and success.
- Get link
- Other Apps